In 2018, over 1.8 million acres burned in California, causing $11 billion in damage. It was a once-in-a-lifetime year for wildfires. Then so was 2020. In fact, fifteen out of the twenty largest wildfires in California history have happened in the last twenty years. And the problem isn’t going away.
With the effects of climate change, wildfires are increasing in frequency and intensity. Beyond the very real environmental destruction and human effects of lost homes and lives, this has also caused a huge disruption in the insurance industry.
The losses are forcing underwriters to rethink their models and assumptions to find a way to properly underwrite this risk. Often they simply can’t. This is mostly because their current models are based on historical data that isn’t accurate anymore. The result is reinsurers increasing premiums astronomically or attempting to back out of markets altogether, creating a coverage vacuum. This has had ripple effects throughout California, with primary home insurers then dropping policies, which down the line could even cause issues with getting a mortgage.
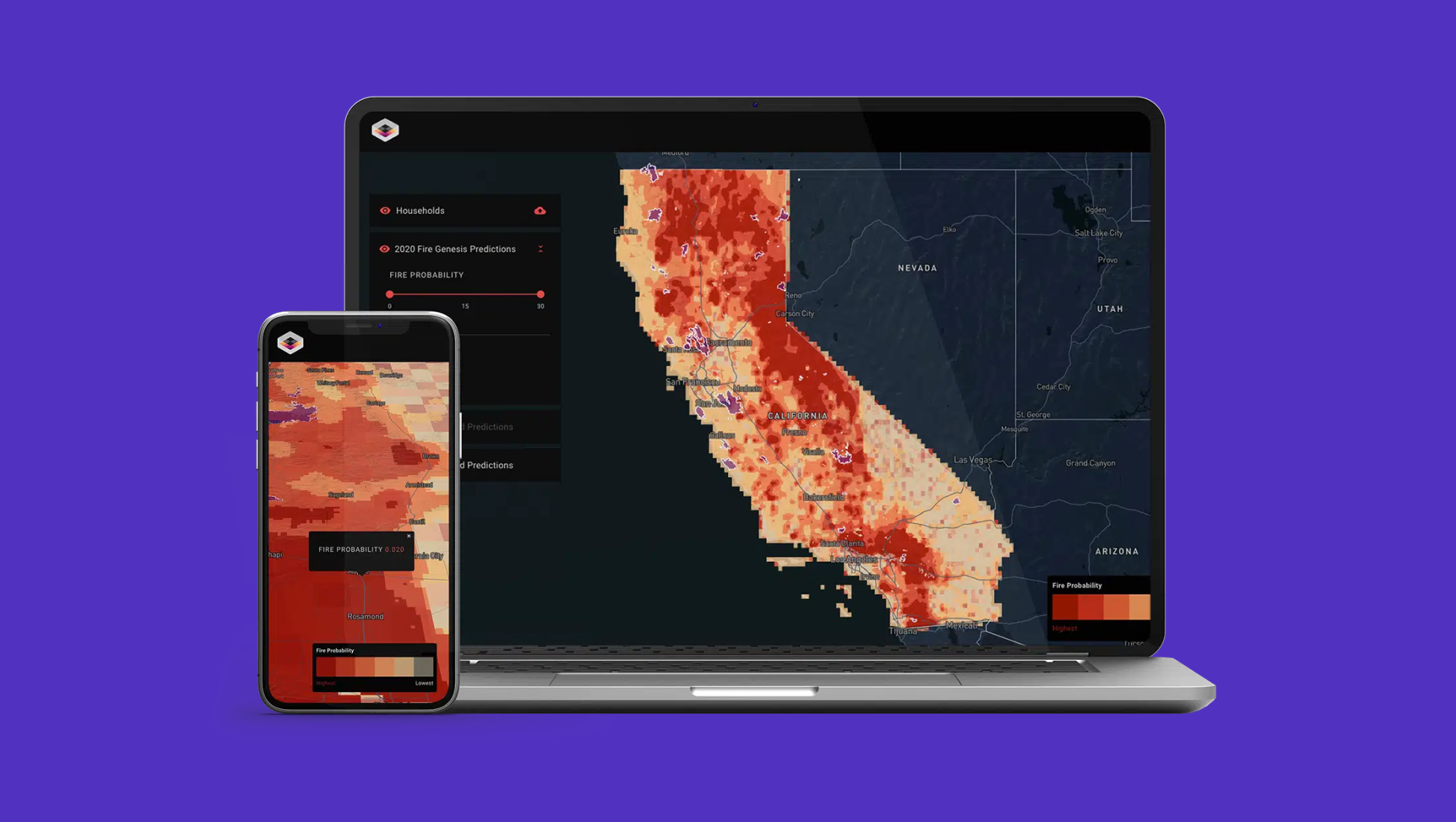
The problem with reinsurance
Kettle is stepping into the $10 billion wildfire reinsurance market using a proprietary model that’s able to predict the occurrence, spread, and severity of wildfires with a much higher degree of accuracy than the rest of the industry.
“It feels like the whole state’s burning down,” says Nat Manning, COO of Kettle. “Last year, 4% of the state acreage did burn, but that was only 11,466 structures out of 14 million. That’s terrible for those homes – but from a data perspective it’s a tenth of a percent.”
The problem, Nat points out, is that current insurance models can’t predict which tenth of a percent are in trouble, so they’re charging as if a quarter of the state is going to burn down. If you could build a model that was more accurate, the trickle down effects would be huge.
“There’s been a 60% drop in the return on equity for the reinsurance industry over the past 10 years,” says Nat. “They’re using the same models they’ve been using for the last 30 years. But the world has changed. We realized you could use machine learning and AI to do a much better job predicting risk, which allows you to have better pricing and bring more stability to this market and ultimately better protect people.”
“There’s been a 60% drop in the return on equity for the reinsurance industry over the past 10 years. We realized you could use machine learning and AI to do a much better job predicting risk.”
Nat Manning
Kettle COO
Finding talent with the right DNA
The Kettle team knew they needed the right technical talent and needed them to start in days, not months.
“We needed our model to be as good as it could possibly be and we were on a timeline, needing to write the most accurate policies we could for the upcoming year,” says Nat. Tribe worked with the Kettle team to design an approach and put together the right talent to execute on it quickly.
“We talked to their team and got a better understanding of their needs – computer vision, integrating disparate data sources and building unique models,” says Jaclyn Rice Nelson, Tribe co-founder.
For many teams, this would mean reaching into their rolodex for insurance specialists. Tribe approaches problems more strategically. “We’re solving problems that have never been solved before so it’s often not a question of bringing on someone who has done that exact thing in that exact industry,” says Jaclyn. “It’s about bringing people who have the right DNA to solve that problem.”
In other words: working on an insurance problem doesn’t mean you use insurance specialists. So the team brought on Adrian, an engineer who formerly worked on Google Earth Engine and has deep satellite imagery expertise, and Gabe, a Stanford CS graduate and ML researcher with expertise in computer vision.
“The Tribe folks really came in and were fully integrated in our team. Adrian was on Slack asking questions, sharing research. It was a benefit for us, having another great, smart voice in the room. He’d been at Google Earth Engine. He had a deeper expertise when it comes to satellite imagery and computer vision than what we had on the team.”
“There was very little precedent for doing this kind of fire modeling or machine learning. So we were really going into the wilderness and trying to achieve something novel.”
Gabe
Tribe machine learning researcher
Updating a legacy industry with AI
Building on Kettle’s existing model, Tribe worked closely with the Kettle team to improve the performance and accuracy. The team approached the challenge by splitting it into two different models: ignition – the likelihood a fire will start at any given location – and contagion – how that fire will spread. The ultimate goal was to try and understand the probability that a specific address (or latitude and longitude) will burn down in a wildfire in the next 12 months.
“There was very little precedent for doing this kind of fire modeling or machine learning,” says Gabe. “There are very few papers that try to do this and historically they haven’t achieved very good results. So we were really going into the wilderness and trying to achieve something novel.”
In their research, the engineers found that past attempts were more reactive. They tended to be based on conditional situations: if a fire starts now, what will happen in the next 24 hours?
“The status quo for modeling in the industry is all based on a couple numbers – how long and wide the fire is today and how it is at the end of the burn,” says Adrian. “They don’t use machine learning or satellite imagery. And they treat every fire like an ellipse – long and narrow – which is not how fires behave, in reality. So everything is based on a couple numbers rather than looking at the big picture.”
The Kettle team, on the other hand, needed to model what would happen months in advance so they can predict risk for the entire year. And they weren’t only trying to model one-step growth, but to predict the entire burn.
The machine learning solution
Gabe and Adrian experimented, each trying a different approach, testing them and sharing the results. “My approach brought components of biomedical research and imaging, where you have to highlight areas of the images,” says Gabe.
“Mine was more intuition based,” says Adrian. “I tried to think about rules that would govern how fires behave and design the model to enforce those rules, then learn as well as it could within those constraints. “The model we built uses data – like satellite imagery – that shows where previous fires actually went. This lets us train the model to find the underlying behavior of fires, rather than assuming every fire behaves as an idealized ellipse.”
“There’s a lot of inherent randomness in fire – a lot of different ways a fire can go,” says Adrian. “And we’re working a year in advance of the next fire season, so what we’re really doing is enumerating all the possibilities. In the optimistic case, the fire will die here. In the worst case, it’s going to jump over to this forest here. The point isn’t saying which will happen definitively, but giving each an accurate probability.”
Tribe’s work with Kettle more than doubled the performance of the existing spread model – which was already far above the industry average. The results have the potential to change the reinsurance industry.
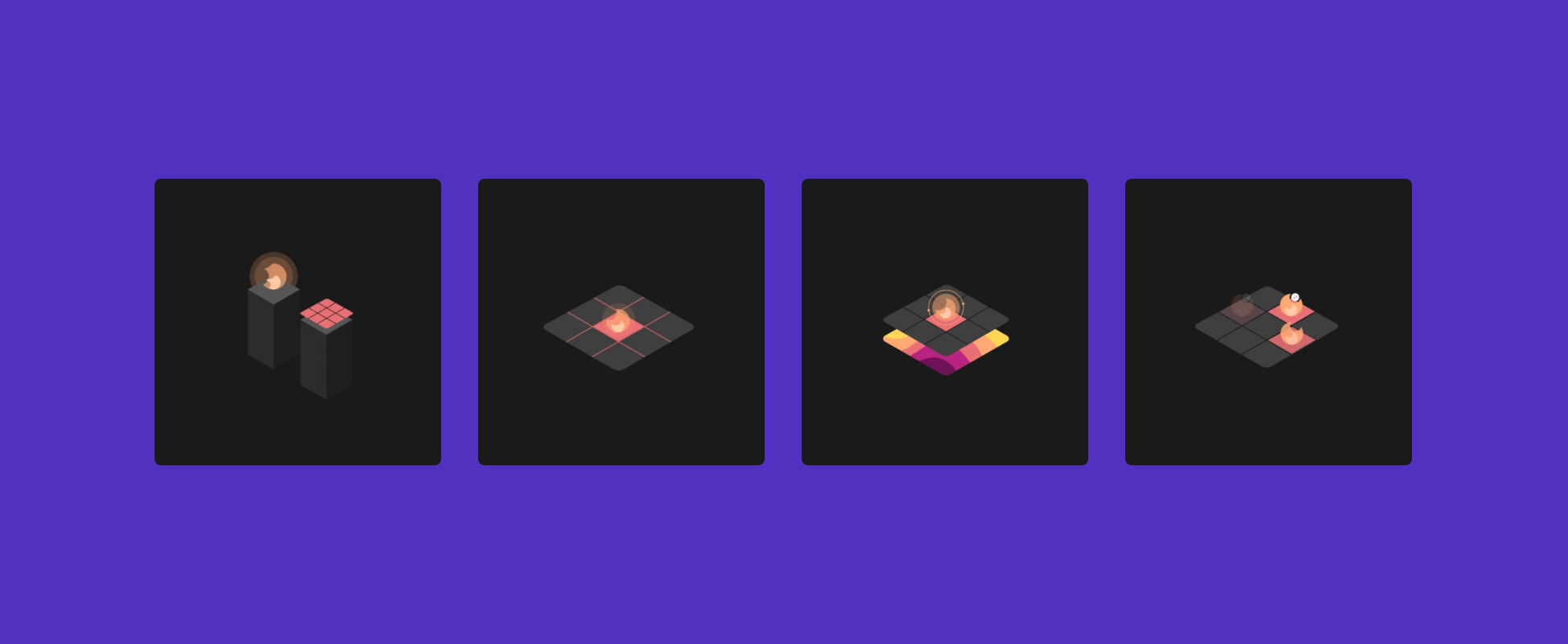
Partnering for impact
For the Kettle team, working with Tribe allowed them to get immediate access to the expertise needed to improve their model, while still growing their in-house team.
“Working with Tribe wasn’t a replacement for building our own team – it was an enabler,” says Nat. “We were able to start working with an engineer right away who had exactly the experience with geospatial data we were looking for. Then, when they turned out to be a great fit, we were able to hire them as an employee. In the end, we shipped faster and hired exactly the right person for the role. It’s the definition of win win.”
And partnership goes both ways. Adrian had the opportunity to work on an interesting problem with real-world impact. And, when the company turned out to be a great fit, he came on as a part time employee.
"The work informs insurance pricing, connects the economy to the cost of climate change, and helps fight active fires,” says Adrian. “A lot of tech companies do mental gymnastics to come up with how they're changing the world, so it's nice to do work where the impact is so clear."
“I took a part time role because I wanted to truly be part of the company – have equity and help make decisions around strategy, while protecting a certain amount of my time to work on other projects.”
“Working with Tribe wasn’t a replacement for building our own team – it was an enabler. In the end, we shipped faster and hired exactly the right person for the role. It’s the definition of win win.””
Nat Manning
Kettle COO
The Impact
“Every year there are over 10,000 wildfires a year in California, but in 2020 fourteen of them caused 99% of the damage,” says Nat. “Until now, no one has been able to account for the nonlinear events that cause a fire to get out of hand. Our approach does a better job predicting how all these factors come together to create these catastrophic level events.”
“It’s exciting on so many levels,” says Jaclyn. “These are actual lives. These are actual people. If Kettle can better predict where the fire is going to spread, the impact is enormous – and not just from a business perspective.”
“Our mission is to balance risk in a changing climate,” says Nat. “At its heart, insurance is about humans cooperating at large scale to share risk. And, in the 21st century, we have new risks. There’s been a threefold increase in billion dollar catastrophes in the last fifteen years in the United States – almost all of them climate change related. Reinsurance is the last backstop for helping people rebuild after these events. Ultimately, what we’re building is a safety net.”
“These are actual lives. These are actual people. If Kettle can better predict where the fire is going to spread, the impact is enormous – and not just from a business perspective.”