AI is taking the world by storm, and businesses across industries are eagerly adopting artificial intelligence tools to automate both internal and consumer-facing processes.
But can an industry as heavily regulated as banking and finance also benefit from these novel technologies?
The short answer is: yes. (The long answer is… well, this whole article.)
According to The Economist, a staggering 85% of IT executives in the banking industry already have a clear strategy for adopting AI in the development of new products and services — and 80% of senior executives in the space believe that successful adoption will distinguish “winners” from “losers.”
Keep reading to find out how, exactly, winning banks and financial institutions are benefiting from generative AI, machine learning, and data science. We’ll also provide tips for safely navigating industry regulations and common risks associated with the early adoption of AI.
Why do banks and financial services companies need AI?
Some of AI’s most widely adopted business uses across industries include:
- automating repetitive tasks and streamlining operations
- organizing complex data and generating insights to improve decision-making
- providing customers with real-time personalized support
- simplifying content creation for marketing purposes
Note how all of these are industry-agnostic. This means that financial institutions could benefit from them just as much as businesses from other verticals. Simply put, if your business has customers, you will benefit from AI automation regardless of whether you’re a bank, a retail chain, a fitness center, or a SaaS company — and so on.
That said, banks and financial institutions are unique in many ways. They handle vast volumes of complex and sensitive financial data. The ways that this information can (and cannot) be handled are heavily regulated. And any technology used in the financial services industry needs elaborate safeguards against fraud. The good news is that with the right AI tools, banks can automate their work and stay safe and compliant.
7 uses for AI in banking and finance
1. Internal operations
AI can streamline internal operations in the financial services industry in many ways. There’s an AI solution for every internal team and function, including:
- Document processing and data entry
- Project management
- Human resources and talent management
- Expense management
- Vendor and supplier management
What would this look like in practice?
Popular project management dashboard Notion, for example, recently introduced an AI “thought partner.” The technology has been embedded into the familiar UI of Notion’s dashboard to help users summarize messy notes, extract follow-up tasks, formulate key takeaways, and more.
And here’s a more specialized example that the Tribe AI team developed in partnership with workforce development platform Bright: an AI-driven chatbot for corporate training.
Bright develops realistic training scenarios that mimic authentic workplace situations. However, the process of manually setting up immersive learning experiences for clients with various needs is a time-consuming, difficult to scale process.
The AI chatbot built to address this challenge is able to adopt various customer personas to enrich training scenarios at scale. It performs three key roles:
- Dynamically generates customer simulations
- Dynamically assists with CSR evaluation in real-time
- It’s a passive, real-time CSR knowledge assistant
2. Marketing
Many marketers have been worried that AI will take their jobs. While it’s a bit early to fully discard this grim notion, we believe that (at least in 2023), AI is more likely to make marketers’ jobs easier and unlock opportunities to do more and better — build a deeper understanding of target audiences, get more campaigns off the ground, automate testing, and drive better overall results. This applies to all industries, and FinServ is no exception.
Let’s take a look at two marketing-specific use cases for AI: market research and content creation.
AI can simplify market research by making sense of data collected through social listening and brand monitoring tools plus reviews, customer interviews, surveys, and more. This could mean evaluating public sentiment, identifying common themes in reviews and online conversations, extracting action items, and even drafting ideas for new banking services. AI tools can also efficiently and effectively perform competitive research. Finally, through the use of predictive analytics, artificial intelligence can flag emerging trends or changing market dynamics.
With all these insights handy, marketers can use generative AI tools to create targeted assets and campaigns in a fraction of the time it would take them without the help of artificial intelligence.
Here’s an example of how marketers can work with ChatGPT to create content for social media:
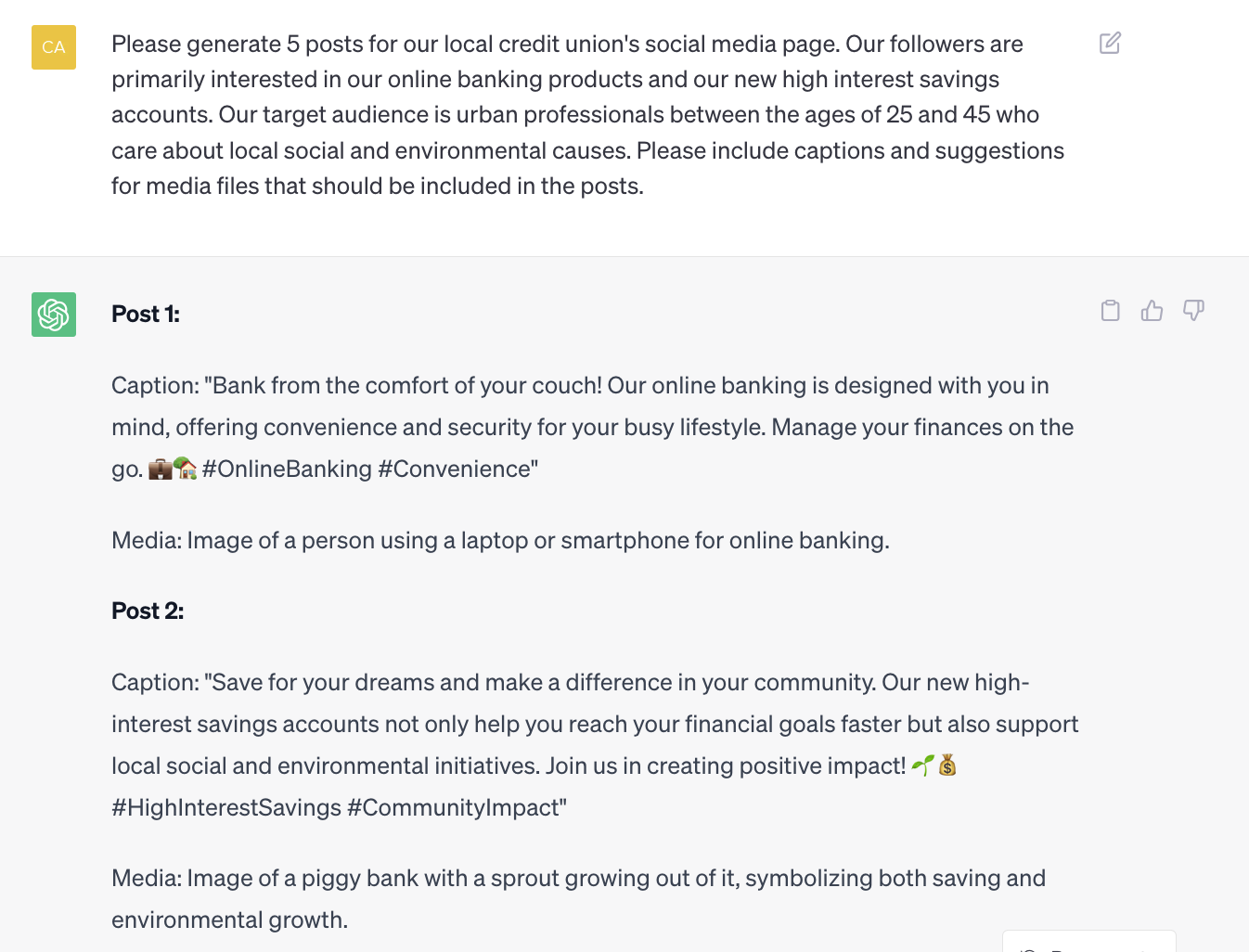
Image: ChatGPT
3. Customer service
AI-powered customer service tools can help protect your institution’s resources and reputation while significantly improving the quality of your customers’ online interactions with your brand.
With artificial intelligence and virtual assistants, you can offer support that’s:
- Live 24/7
- Available in all of the languages your customers speak
- Personalized
- Designed to resolve issues and promote new products and services
But AI-powered support tools don’t only serve customers — they also simplify the work of human agents by automating message routing, extracting conversation summaries, saving customer insights for future reference, and analyzing all customer service conversations for common topics.
Here’s what an AI-powered customer insights dashboard from Microsoft Dynamics looks like:

4. Data analysis
In the ever more saturated banking sector, AI can help organizations make the right decisions based on data. AI systems process data quickly and at scale, turning raw information into actionable insights (and detailed visualizations) for decision-makers, and helping institutions stay relevant and competitive.
It’s important to note that AI’s ability to process vast and unstructured datasets helps businesses with both short and long-term strategies. Artificial intelligence can be used to power advanced predictive analytics tools that formulate reliable forecasts — about market trends, consumer behaviors, etc. — based on available historical data.
5. Fraud detection
Artificial intelligence systems can also play a critical role in identifying and preventing fraud by uncovering unusual behaviors and data patterns in real time.
AI's strength lies in its ability to spot the most subtle deviations, even in complex financial transactions. Through machine learning, AI learns from past data to differentiate legitimate actions from fraudulent ones, enhancing its accuracy over time. This continuous learning process allows AI to stay ahead of evolving fraud and money laundering tactics — and keep both the assets and the reputations of financial institutions safe.
This is what AI-powered risk assessment insights look like in Hawk AI’s transaction fraud detection dashboard:
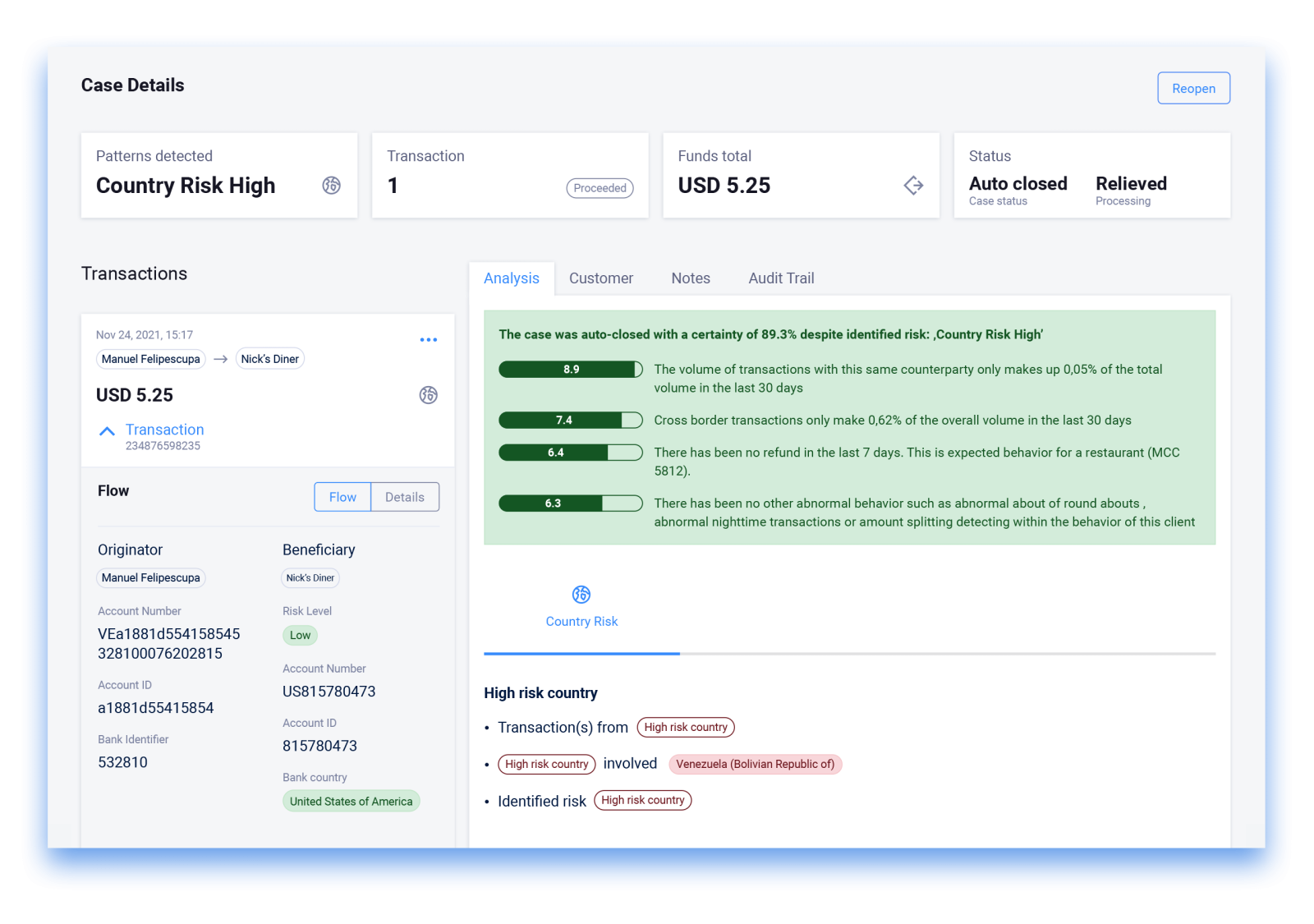
6. Credit scoring
Traditionally, credit scoring relied on credit bureaus assessing the likelihood that an individual would repay a loan based on data from various (but solely finance-related) sources: credit reports, payment histories, outstanding debts, etc.
AI can enhance this process by taking a broader range of data sources — including non-traditional information like transaction behaviors and online activities — into account. It also promotes fairness and ensures more equitable access to credit by removing the potential for bias that often comes with human judgment. In general, AI-driven credit scoring offers a more comprehensive, accurate, and inclusive assessment of borrowers' creditworthiness.
7. Regulatory compliance
Financial services is a heavily regulated industry. AI-powered tools can automate compliance monitoring and reporting processes by analyzing regulatory requirements, monitoring business operations, flagging compliance risks, and generating reports.
Traditional regulatory compliance involves manual efforts by compliance officers to interpret regulations, cross-reference policies, and conduct audits. While AI tools are not likely to completely replace human auditors, they can streamline these processes by automating the analysis of regulations, cross-referencing them with internal policies, and providing real-time alerts for changes.
The benefits of using AI in banking and finance
Across all the use cases listed above, these are the most important benefits you can expect to gain from your investment in AI.
- Better insights. AI can process and analyze vast amounts of data at scale, providing human analysts with essential insights and visualizations, and helping executives make informed decisions. This benefit is also crucial in the context of risk management and fraud detection.
- Improved customer experience. Using AI to provide customers with 24/7 personalized support can significantly improve customer satisfaction.
- Efficiency and time savings. Automating repetitive tasks and streamlining internal processes can help financial institutions optimize resourcing and operational costs.
- Simplified compliance. AI monitoring (of regulatory announcements as well as your institution’s processes and outbound communications) helps avoid penalties and reputational damage.
- Automated market intelligence. AI's predictive capabilities can help organizations stay up to date with market trends and consumer expectations — and automate the process of identifying investment opportunities.
- Competitive edge. Innovating operations and reacting to market expectations with agility will improve your institution’s overall competitiveness.
The risks of using AI models in banking and finance
The adoption of AI tools yields significant benefits for banks and FinServ companies, but it also comes with risks that institutions must carefully manage to ensure data security and regulatory compliance.
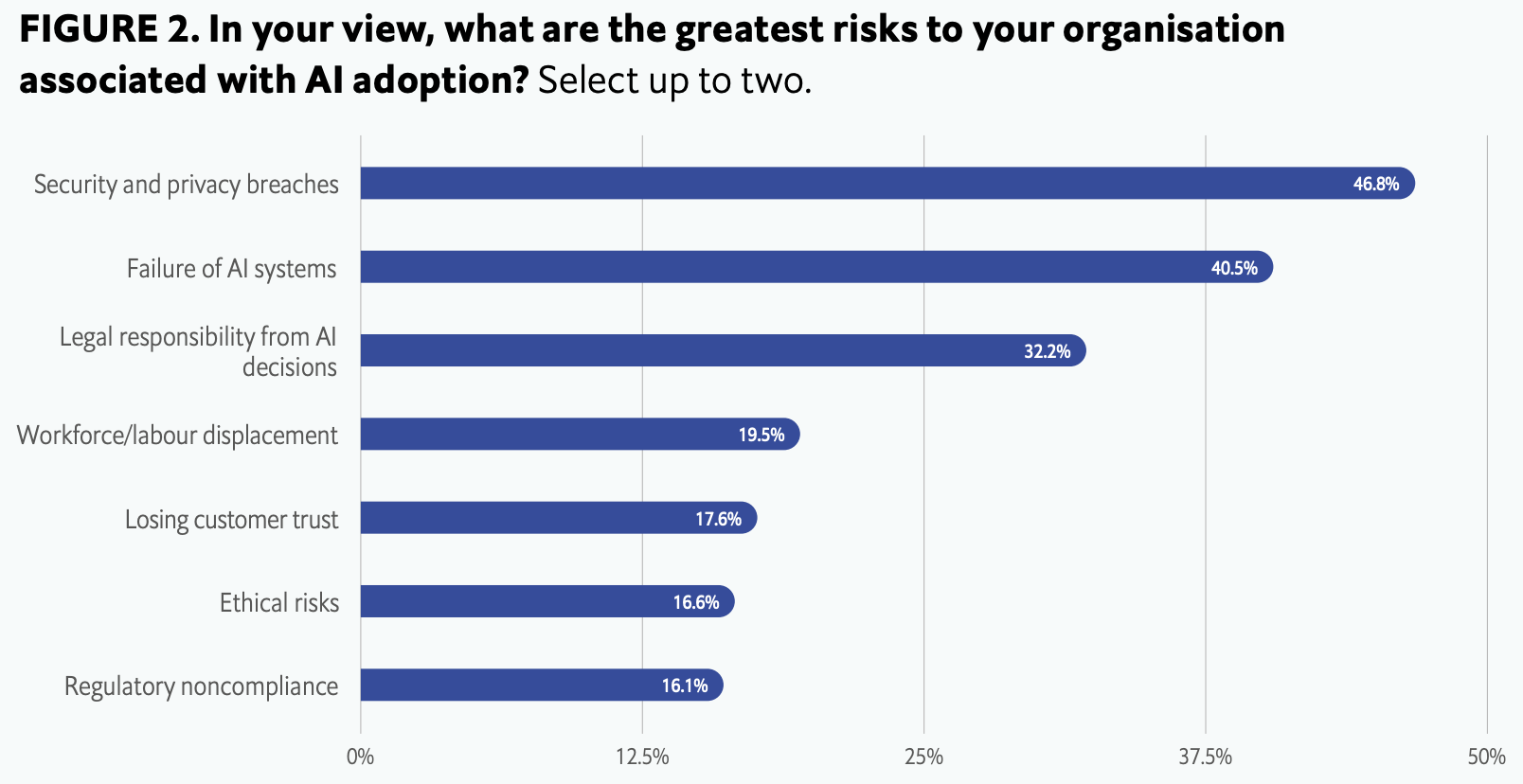
According to a survey conducted by The Economist:
“[...] firms are treading carefully, balancing business benefits against regulatory complexity and the need to maintain customers’ trust. Most banks (62%) agree that the complexity and risks associated with handling personal data for AI projects often outweigh the benefits to customer experience.”
Here are some key risks associated with using AI in this sector:
Data security and privacy risks
- Data breaches. AI systems with access to large amounts of sensitive customer data can become attractive targets for hackers — and any breach could lead to the exposure of personal and financial information, compromising the clients’ safety and the institution’s reputation.
- Data misuse. Without a solid implementation strategy that includes proper security measures, unauthorized individuals could access, misuse, or manipulate customer data.
Regulatory compliance risks
- Data privacy regulations. Organizations must ensure that their use of AI technology adheres to global and local data privacy regulations (e.g. the General Data Protection Regulation and the California Consumer Privacy Act), which impose strict requirements on data collection, storage, and processing.
- Financial regulations. AI systems used for credit scoring, risk assessment, and investment decisions must also comply with financial regulations to ensure fairness, transparency, and prevention of discriminatory practices.
Biased algorithms
- Bias in data. If the data used to train LLMs and AI algorithms is biased, the systems can produce discriminatory outcomes. In financial services, this can potentially affect credit scoring and lending decisions — and trigger legal and reputational issues.
Operational risks
- Model reliability. AI models that are not thoroughly tested may generate incorrect predictions or recommendations, leading to misguided decisions.
- System downtime. Heavy reliance on AI-powered systems can lead to operational disruptions in the event of system failures. (However, this is also true for technology used in financial services that does not utilize artificial intelligence.)
- User error. Since artificial intelligence for business is relatively new, end users require thorough training for organizations to access the full value of AI solutions.
Ethical concerns
- Job displacement. Automation driven by AI could lead to workforce reduction, sparking ethical concerns about job losses and societal impact.
- Unintended consequences. AI-driven decisions might have unintended consequences, such as altering market dynamics or influencing consumer behaviors in unexpected ways.
Legal risks
- Legal interpretation. Determining legal liability for misguided decisions can be very difficult when mistakes or harms are a direct outcome of decisions based on insights generated by AI algorithms.
So, how can financial institutions navigate and mitigate these risks?
First of all, seek the guidance of industry experts when planning, implementing, and monitoring the work of AI systems. Working with an experienced team of consultants will help you gain a competitive edge while staying compliant, sustaining ethical standards, and upholding legal obligations specific to your industry.
You might also want to start slow. To set up clear implementation processes, test systems for security, and get stakeholder buy-in, focus on internal use cases first. This could mean using AI for employee training and onboarding, setting up an internal chatbot to help teams navigate company policies, or giving your marketing team access to AI-powered content generators. Once you've internally proven the feasibility and value of AI, you can move on to more complex customer-facing solutions with more confidence.
Bring your bank into the future with AI
Artificial intelligence tools are helping financial institutions cut operational costs and optimize profits while improving the quality of their services and customer experience.
Wondering how you can embrace AI to stay competitive and relevant?
Tribe.ai is an expert AI consulting company that connects businesses with industry-leading experts and data scientists. Our team is here to guide you all the way from ideation to implementation. Contact us to book a free consultation and find out how AI can help your institution.